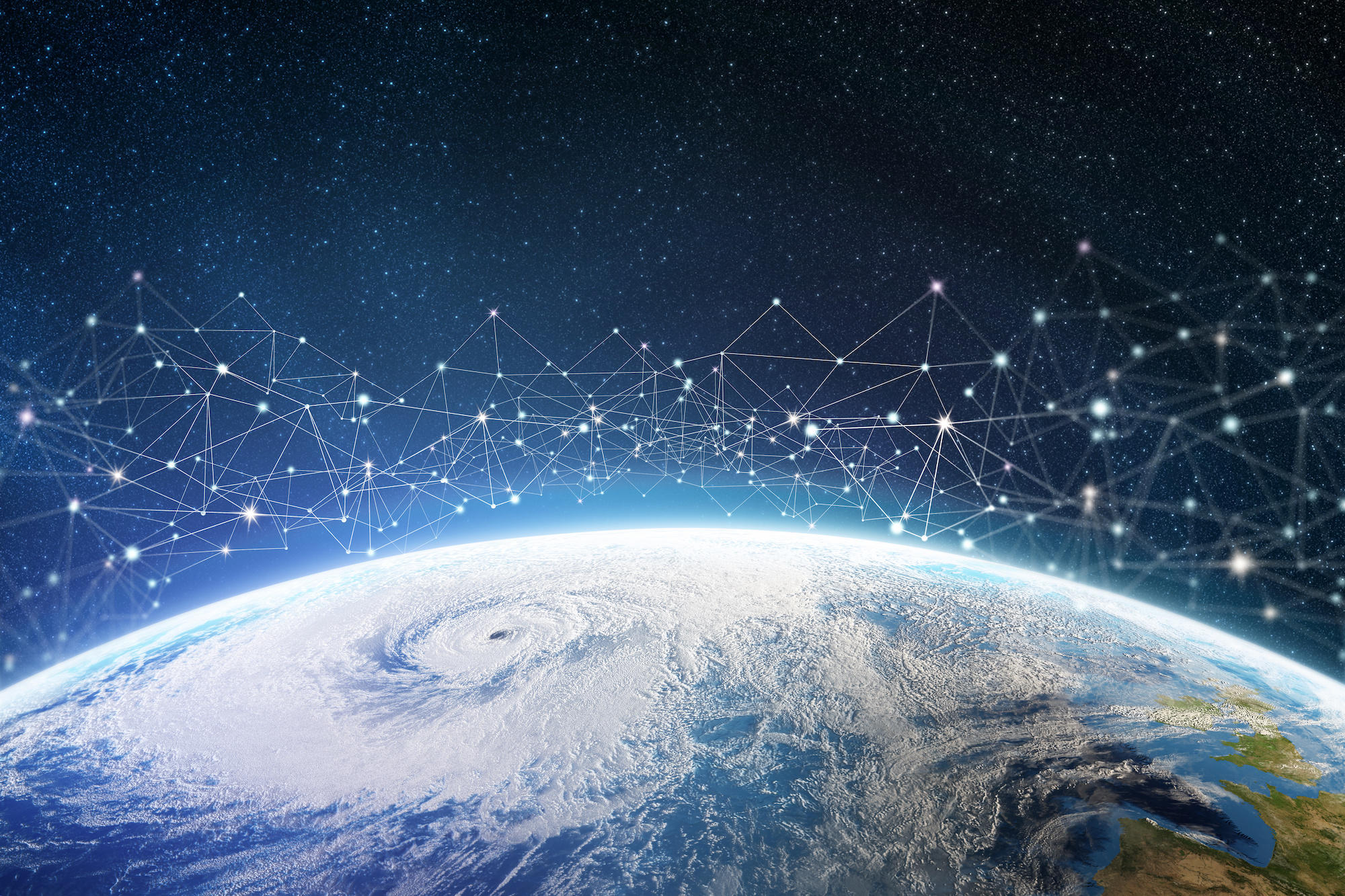
About Me
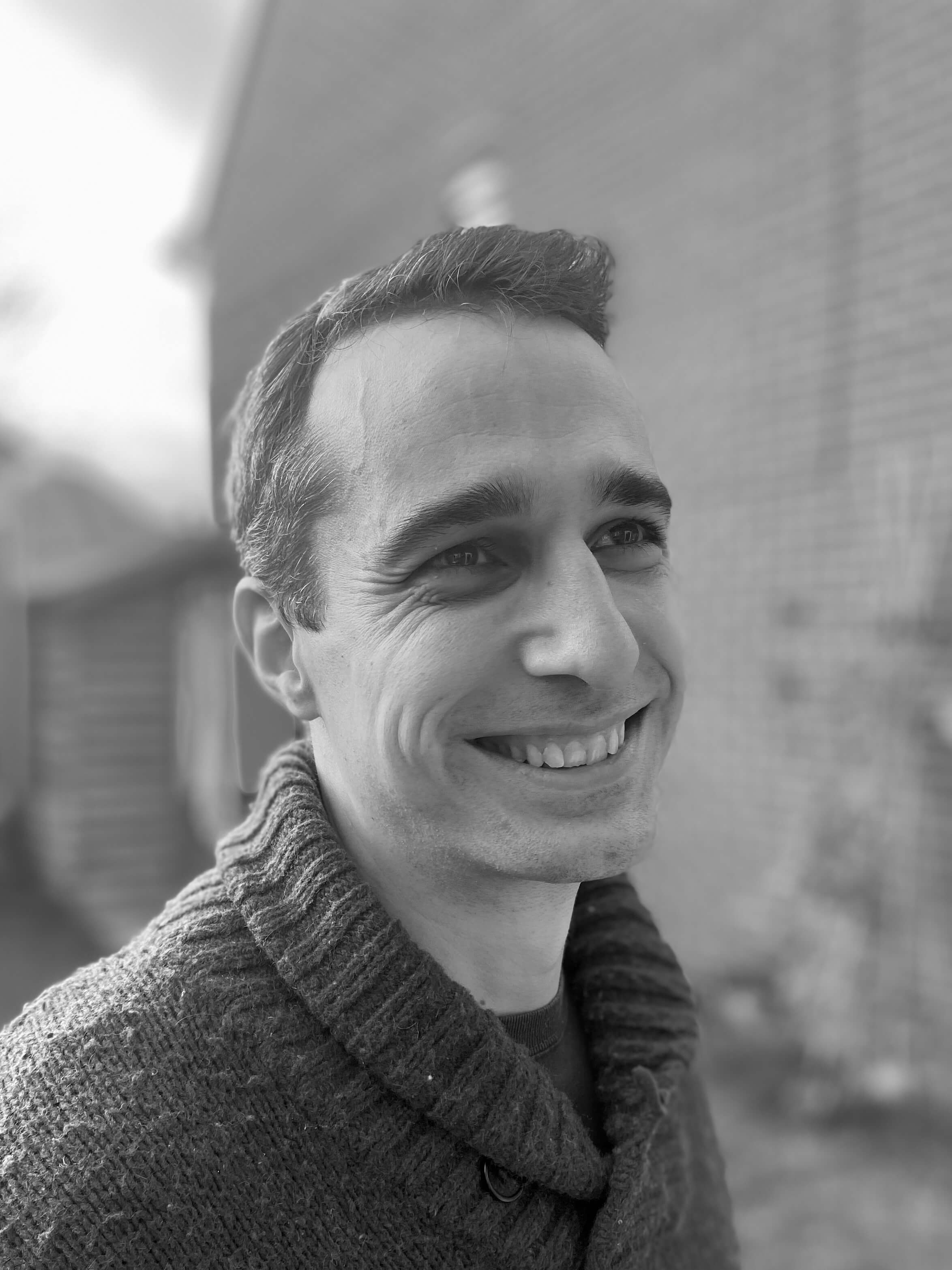
I'm an atmospheric physicist working at the interface of climate research and machine learning. The Climate Analytics Lab I lead focuses on understanding the interactions between aerosols and clouds, and their representation within global climate models. These interactions are numerous and complex, involving non-linearities and feedbacks which make modelling average responses to any perturbation in aerosol extremely challenging. We're leading the development of a variety of machine learning tools and techniques to alleviate these difficulties and optimally combine a variety of observational datasets, including global satellite and aircraft measurements, to constrain and improve these models.
I'm also keen to foster the application of machine learning to climate science questions more broadly and convene the Machine Learning for Climate Science EGU session and co-convene the “AI and Climate Science” discovery series that is part of the United Nations’ AI for Good program.
Recent work
A selection of recent projects I've been working on; a more comprehensive list can be found under Projects.
In the News
This article nicely describes the counter-intuitive effect that aerosol have on the climate, and in particular their importance for reducing uncertainty in future warming as revealed in our Nature Climate Change paper.
This article in The Conversation describes the need for urgent reductions in fossil fuel burning in light of the larger than expected cooling effect of aerosol on the climate, as highlighted in our recent work on detecting ship-tracks.
This article from the Wall Street Journal explores the role of Amazon, Microsoft and Google in meeting the need for cloud-scale computing to tackle some of the largest climate research problems we face. It highlights my work on detecting ship-tracks in vast quantities satellite imagery using Machine Learning on Amazon Web Services.
In this podcast Philip Stier and I discuss the use of cloud computing for tackling some of the most pressing climate change questions, including for hosting large machine learning workflows such as the detection of pockets of open cells. This was also featured in a blog post by Amazon CTO Werner Vogels.
This article in Science magazine highlights our work using neural architecture search to develop fast, flexible emulators that are applicable to a wide range of physical simulators. These emulators are often orders of magnitude faster than the simulators they replicate and can allow solving inverse problems - that is, determining model inputs based on matching observable outputs.